每年Gartner发布的技术成熟度曲线(The Hype Cycle)都备受市场关注,也成为企业精准创新、重大投资决策的风向标。今年新兴技术成熟度曲线最亮眼的就是人工智能技术。基于数据,人们可以解决超乎想象的问题。不少朋友在问企业hands on深度学习的人工智能怎么做,我来给大家做一个关于编码细节程度的卷积神经网络、基于MNIST数据集的实验,带您一探究竟。
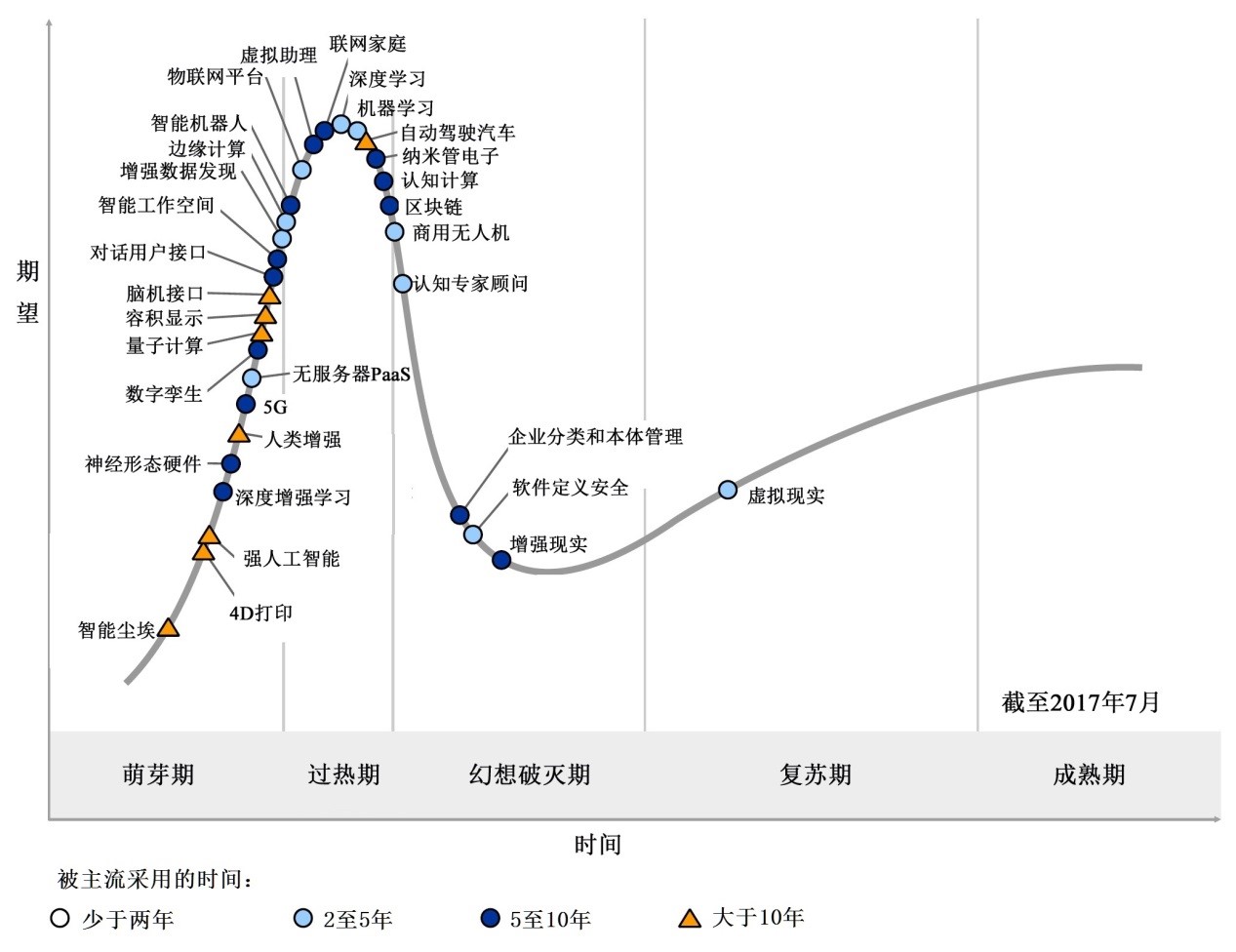
未来10年人工智能将成为最具颠覆性的技术,主要源于越来越强的计算能力、越来越海量的互联网和物联网数据以及深度学习理论的快速进步。
深度学习研究的热潮持续高涨,各种开源深度学习框架也层出不穷,其中包括Google的TensorFlow、Keras、Facebook的Caffe、Torch、Microsoft的CNTK、Amazon的MXNet、以及蒙特利尔大学的Theano等等。而现在几乎所有主流云平台都支持深度学习框架,使用框架创建神经网络就变成一件轻而易举的事情了。
下面简单演示一下使用pyTorch创建一个卷积神经网络,使用经典的MNIST数据集进行训练和测试。
我们知道:创建一个神经网络主要有4个步骤:
- 定义神经网络的结构
- 定义损失函数
- 在会话中,将数据输入进构建的神经网络中,反复优化损失函数,直至得到最优解
- 将测试集丢入训练好的神经网络进行验证
PyTorch中定义卷积神经网络
class CNN(nn.Module):
def __init__(self):
super(CNN, self).__init__()
self.conv1 = nn.Sequential( # input shape (1, 28, 28)
nn.Conv2d(
in_channels=1, # input height
out_channels=16, # n_filters
kernel_size=5, # filter size
stride=1, # filter movement/step
padding=2, # if want same width and length of this image after con2d, padding=(kernel_size-1)/2 if stride=1
), # output shape (16, 28, 28)
nn.ReLU(), # activation
nn.MaxPool2d(kernel_size=2), # choose max value in 2x2 area, output shape (16, 14, 14)
)
self.conv2 = nn.Sequential( # input shape (1, 14, 14)
nn.Conv2d(16, 32, 5, 1, 2), # output shape (32, 14, 14)
nn.ReLU(), # activation
nn.MaxPool2d(2), # output shape (32, 7, 7)
)
self.out = nn.Linear(32 * 7 * 7, 10) # fully connected layer, output 10 classes
def forward(self, x):
x = self.conv1(x)
x = self.conv2(x)
x = x.view(x.size(0), -1) # flatten the output of conv2 to (batch_size, 32 * 7 * 7)
output = self.out(x)
return output, x # return x for visualization
下面使用MNIST数据集训练网络。MNIST 数据集来自美国国家标准与技术研究所,National Institute of Standards and Technology (NIST)。训练集 (training set) 由来自 250 个不同人手写的数字构成,其中 50% 是高中学生,50% 来自人口普查局 (the Census Bureau) 的工作人员。
测试集(test set) 也是同样比例的手写数字数据。MNIST 数据集可在 http://yann.lecun.com/exdb/mnist/ 获取。
# Mnist digits dataset
if not(os.path.exists('./mnist/')) or not os.listdir('./mnist/'):
# not mnist dir or mnist is empyt dir
DOWNLOAD_MNIST = True
train_data = torchvision.datasets.MNIST(
root='./mnist/',
train=True, # this is training data
transform=torchvision.transforms.ToTensor(), # Converts a PIL.Image or numpy.ndarray to
# torch.FloatTensor of shape (C x H x W) and normalize in the range [0.0, 1.0]
download=DOWNLOAD_MNIST,
)
# plot one example
print(train_data.train_data.size()) # (60000, 28, 28)
print(train_data.train_labels.size()) # (60000)
plt.imshow(train_data.train_data[0].numpy(), cmap='gray')
plt.title('%i' % train_data.train_labels[0])
plt.show()
# Data Loader for easy mini-batch return in training, the image batch shape will be (50, 1, 28, 28)
train_loader = Data.DataLoader(dataset=train_data, batch_size=BATCH_SIZE, shuffle=True)
# convert test data into Variable, pick 2000 samples to speed up testing
test_data = torchvision.datasets.MNIST(root='./mnist/', train=False)
test_x = Variable(torch.unsqueeze(test_data.test_data, dim=1), volatile=True).type(torch.FloatTensor)[:2000]/255. # shape from (2000, 28, 28) to (2000, 1, 28, 28), value in range(0,1)
test_y = test_data.test_labels[:2000]
运行程序,我们画出第一个数字是5。
一些模块的引入语句如下,然后开始训练网络。
import os
# third-party library
import torch
import torch.nn as nn
from torch.autograd import Variable
import torch.utils.data as Data
import torchvision
import matplotlib.pyplot as plt
# torch.manual_seed(1)# reproducible
# Hyper Parameters
EPOCH = 1 # train the training data n times, to save time, we just train 1 epoch
BATCH_SIZE = 50
LR = 0.001 # learning rate
DOWNLOAD_MNIST = False
下面是测试网络部分代码:
cnn = CNN()
print(cnn) # net architecture
optimizer = torch.optim.Adam(cnn.parameters(), lr=LR) # optimize all cnn parameters
loss_func = nn.CrossEntropyLoss() # the target label is not one-hotted
# following function (plot_with_labels) is for visualization, can be ignored if not interested
from matplotlib import cm
try: from sklearn.manifold import TSNE; HAS_SK = True
except: HAS_SK = False; print('Please install sklearn for layer visualization')
def plot_with_labels(lowDWeights, labels):
plt.cla()
X, Y = lowDWeights[:, 0], lowDWeights[:, 1]
for x, y, s in zip(X, Y, labels):
c = cm.rainbow(int(255 * s / 9)); plt.text(x, y, s, backgroundcolor=c, fontsize=9)
plt.xlim(X.min(), X.max()); plt.ylim(Y.min(), Y.max()); plt.title('Visualize last layer'); plt.show(); plt.pause(0.01)
plt.ion()
# training and testing
for epoch in range(EPOCH):
for step, (x, y) in enumerate(train_loader): # gives batch data, normalize x when iterate train_loader
b_x = Variable(x) # batch x
b_y = Variable(y) # batch y
output = cnn(b_x)[0] # cnn output
loss = loss_func(output, b_y) # cross entropy loss
optimizer.zero_grad() # clear gradients for this training step
loss.backward() # backpropagation, compute gradients
optimizer.step()# apply gradients
if step % 50 == 0:
test_output, last_layer = cnn(test_x)
pred_y = torch.max(test_output, 1)[1].data.squeeze()
accuracy = sum(pred_y == test_y) / float(test_y.size(0))
print('Epoch: ', epoch, '| train loss: %.4f' % loss.data[0], '| test accuracy: %.2f' % accuracy)
if HAS_SK:
# Visualization of trained flatten layer (T-SNE)
tsne = TSNE(perplexity=30, n_components=2, init='pca', n_iter=5000)
plot_only = 500
low_dim_embs = tsne.fit_transform(last_layer.data.numpy()[:plot_only, :])
labels = test_y.numpy()[:plot_only]
plot_with_labels(low_dim_embs, labels)
plt.ioff()
# print 10 predictions from test data
test_output, _ = cnn(test_x[:10])
pred_y = torch.max(test_output, 1)[1].data.numpy().squeeze()
print(pred_y, 'prediction number')
print(test_y[:10].numpy(), 'real number')
运行结果:
话说哪位老铁有多余的有CUDA功能的Geforce显卡,当初入了A家,现在想自己在深度学习领域hands-on一下,有点欲哭无泪啊……